How a Solid Data Strategy Sets the Stage
for Successful Data Cloud & Agentforce Projects
Think about the last time you tried to locate important customer information in your organization. Maybe you needed a quick snapshot of how engaged a client was, only to find yourself chasing metrics through multiple systems—CRM, marketing automation, customer success dashboards, and maybe even spreadsheets or custom databases. Now imagine being able to unify those scattered threads of data, orchestrate them into a clean, consolidated view of each customer, and then layer on the power of AI-driven tools like Agentforce to truly supercharge the way you engage and serve your audience.
This is precisely the promise of Salesforce Data Cloud and Agentforce. However, the road from legacy data chaos to seamless, AI-empowered workflows can be rocky if you ignore one critical element: your data strategy. A well-founded strategy is what keeps teams on track, ensures data is reliable, and protects against hidden pitfalls—whether that’s regulatory compliance issues, runaway usage costs, or plain old “garbage in, garbage out.”
Below, we’ll explore why investing in a thorough data strategy is so vital, and how following a deliberate, methodical approach not only sets the stage for Data Cloud success but also maximizes the potential of Agentforce. You’ll find some bullet points along the way—clear, concise takeaways—but let’s walk through the narrative of how your data can finally become your most powerful asset.
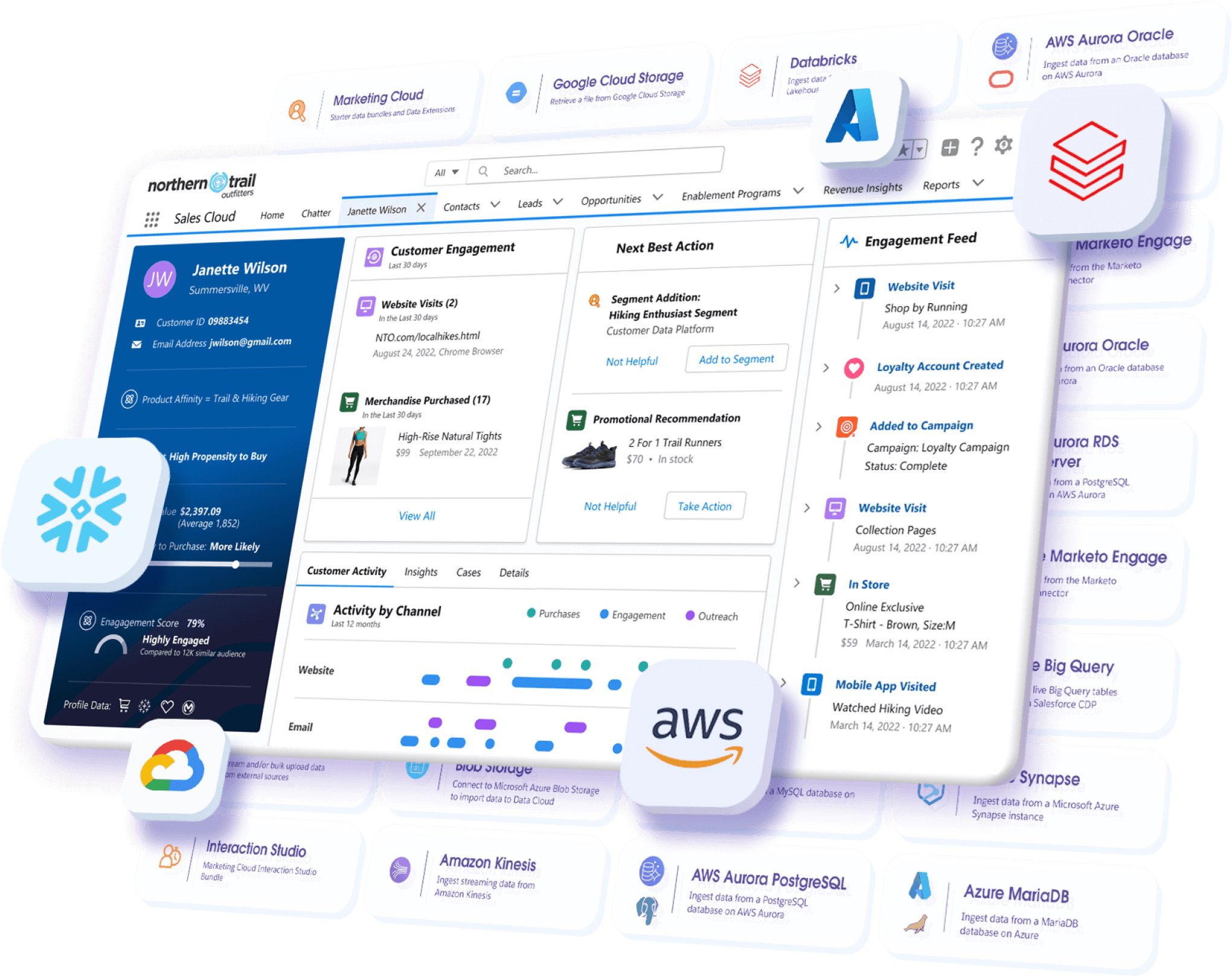
Data Is the Lifeblood of Agentforce: Why Quality Counts
To set the stage, picture Agentforce as your always-on assistant, capable of autonomously handling routine tasks, escalating complex requests, or proactively nudging customers at just the right time. Under the hood, all those fancy agent skills rely on consistent, accurate data that is easy to discover, understand, trust, and access. When we say, “Good data is gold,” it’s not just a cliché. It’s a principle that, when neglected, can derail even the most exciting AI pilots.
So, what does “good data” look like?
-> Discoverable: Agents (and your humans) must be able to locate the data they need immediately, with minimal friction.
-> Understandable: Business context, definitions, and lineage are clear. If your team doesn’t know what a particular field or metric truly represents, data trust plummets.
-> Reliable: Data must be accurate, secure, and compliant so that every decision or AI-driven action stands on a solid footing.
-> Accessible: Instead of buried in a specialized data warehouse or restricted to the department that generated it, valuable information should be available across the organization. Everyone, from marketing to service teams, should be able to pull insights without specialist queries or coding.
When these attributes are missing, the results can be disastrous. Imagine an Agentforce chat skill that repeatedly messages a customer about an old product they no longer use—or, worse, messages the wrong contact entirely because of an outdated record. In the best-case scenario, your new solution is merely confusing. In the worst-case scenario, you lose that customer’s trust entirely.
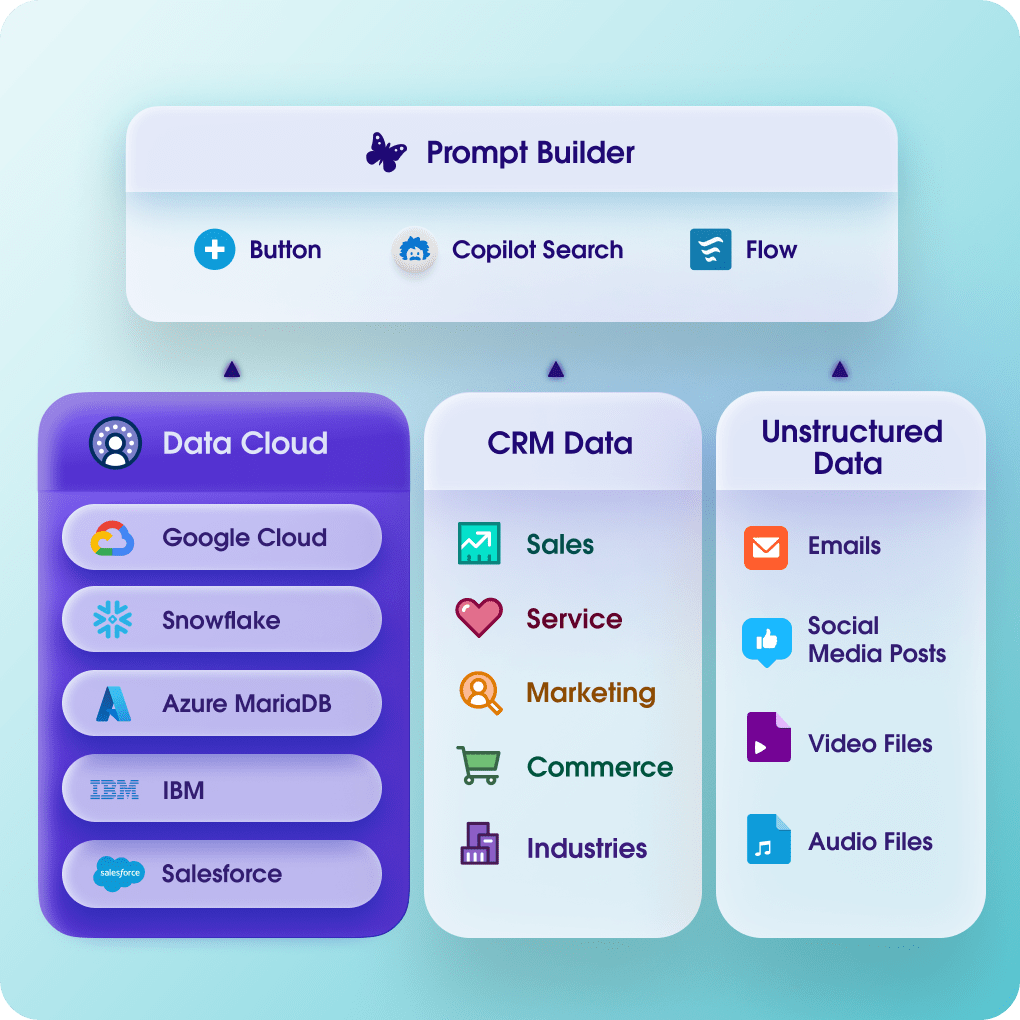
The Hidden Dangers of Bad Data
One Salesforce leader once demonstrated a CRM record that still had them listed at a former company—an all-too-common mistake that underscores the real cost of disorganized data. For an AI agent or chatbot trying to provide relevant messaging and support, incorrect records like these become showstoppers. Not only do they create friction for internal teams, but they can also negatively impact customer satisfaction.
This risk is why a strong data strategy—covering everything from data hygiene and validation to long-term governance—is an absolute prerequisite. It’s not simply a “nice to have”; it’s integral to every step of your project. By addressing potential issues in your underlying data before you integrate new AI-driven solutions, you ensure that each new initiative has a fighting chance of success.
Four Key Best Practices for a Data Cloud–Driven Strategy
Now that we’ve established the imperative for good data, let’s drill into the core pillars of building a robust data strategy that both protects and energizes your Data Cloud journey. While each project will have unique demands, these four best practices consistently emerge across Salesforce “Salesforce on Salesforce” sessions and real-world “customer zero” experiences. Let’s walk through them in a more narrative fashion.
1. Start with Clear Business Use Cases
Far too often, teams jump right into tooling—eager to stand up Data Cloud connectors or spin up new AI agent skills—only to stall out or deliver minimal impact because they never clarified why they needed that data to begin with. The first, most essential step is to pinpoint your core objectives.
- Identify Critical Pain Points: Are customer service teams constantly grappling with incomplete histories? Are marketing teams duplicating outreach because they can’t see unified customer data? Or maybe your sales agents need an intelligent SDR bot that can triage and nurture low-touch leads.
- Quantify the Value: If you’re merging millions of records, does the effort and expense translate to clear ROI? This is crucial for gaining leadership buy-in and preventing scope creep.
- Focus on Tangible Outcomes: Whether it’s building an engagement score or consolidating a single ‘unified individual’ view, each use case should detail exactly what success looks like—e.g., “A 20% reduction in repeated inbound service calls” or “50% faster lead response time.”
When you’re able to say, “This is the bottleneck we want to fix, and here’s how Data Cloud will solve it,” the entire process—from data discovery to final dashboards—becomes more meaningful and results-driven.
Pro Tip: If you aren’t sure where to begin, sit down with the leaders of each business unit—Sales, Service, Marketing, and beyond. Ask them about their biggest data headaches. You’ll likely find that a fresh data strategy can relieve many recurring problems and create powerful AI-driven enhancements.
2. Spend ~90% of Your Time Planning, ~10% Building
It can be tempting to think Data Cloud or Agentforce will be an easy “plug-and-play.” After all, Salesforce often touts a “clicks not code” philosophy, and in many cases, building flows or configuring connectors is indeed streamlined. But the truth is, those are the final steps in a careful, methodical process.
Think of the old woodworking adage, “measure nine times, cut once.” The same logic applies here:
- Architecture & Orchestration: Data Cloud can ingest enormous volumes of data from diverse sources, run identity resolution, and feed all that into Agentforce. But to keep the machine running smoothly, you must thoroughly map out data streams, transformations, and fail-safe steps.
- Data Volume & Governance: Delving into social feeds or call-center transcripts can reveal privacy, compliance, or storage cost questions you must solve up front.
- Org Provisioning & Multi-Org Strategy: If you have multiple Salesforce orgs, deciding whether to centralize Data Cloud or run separate “home orgs” affects how data will be shared and governed later. This architecture choice can’t be taken lightly.
- Data Spaces & Policy-Based Access: Partitioning data for different brands or teams might be easy in theory, but it requires precise rules around who sees what. Proper planning here will prevent a future headache when additional teams want to leverage your Data Cloud assets.
By taking a “measure nine times” approach, you also align different stakeholders, clarify scope, and protect yourself against hidden disruptions. The actual activation of Data Cloud or creation of an Agentforce skill might be just a few quick clicks—but getting to that point responsibly should be 90% of your effort.
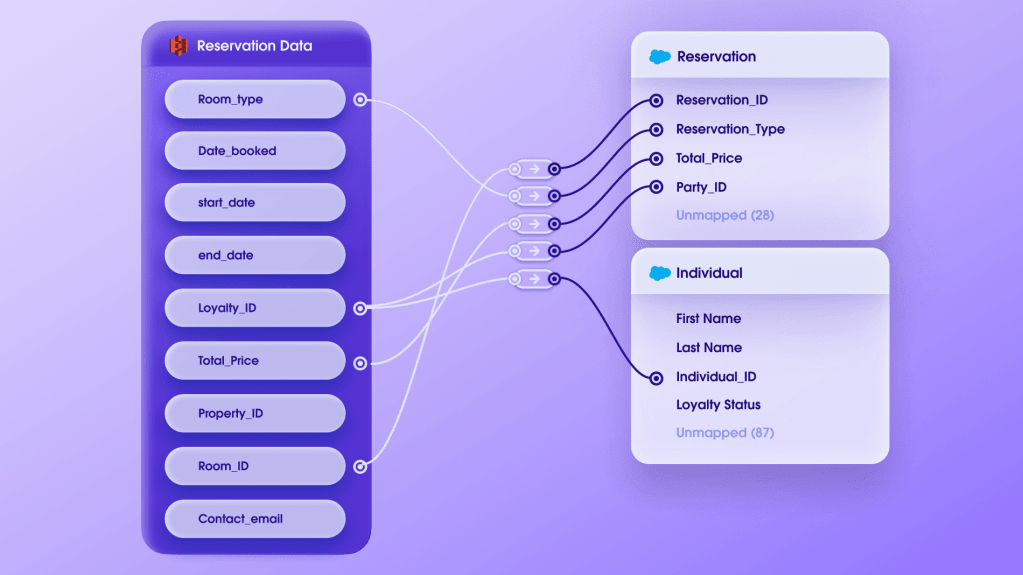
3. Account (and budget) for Consumption-Based Pricing
Whether you’re a longtime Salesforce customer or brand-new to the ecosystem, it’s easy to be surprised by Data Cloud’s usage-based cost model. This is a distinct break from the standard “per user, per month” mindset common in CRM. In Data Cloud, costs accumulate each time you:
- Ingest Data – Whether batch or streaming
- Perform Identity Resolution – Matching and merging records
- Run Queries or Segmentation – For analytics or powering agent recommendations
- Generate or Update Calculated Insights – Which can be invaluable for real-time personalization
The net effect is you can burn through credits faster than you anticipate, especially if you’re loading millions of rows in a sandbox environment for testing. The same caution applies when you roll out an Agentforce skill that frequently queries Data Cloud for real-time updates.
Pro Tip: The best defense against unwelcome surprises is building a cost matrix. List your data sources, expected daily or weekly record volumes, transformation cadence, and key usage scenarios. Multiply by the negotiated cost-per-million for each type of operation. Then compare these to the business value your use case unlocks. Not only does this keep CFOs happy, it also forces you to design usage patterns efficiently.
4. Build a Culture of Documentation & Governance
Now, let’s talk about an often overlooked but absolutely essential pillar: documenting your data flows and dependencies. When you unify data from multiple places—some of them outside Salesforce—understand that a single picklist value in your CRM might ripple through an identity resolution process, a real-time pipeline, and eventually land in a crucial Agentforce skill.
- Avoid Unexpected Breakages: If your marketing team unilaterally changes a field name or your IT team modifies data ingestion rules, how many downstream objects are affected? Without clear documentation, you’re flying blind.
- Scaling Over Time: Your first few Data Cloud use cases may be modest. But as you see results, new requests will come rolling in. Detailed diagrams, process maps, and data dictionaries help new projects get off the ground faster while preserving your existing ecosystem’s integrity.
- Maintain Trust in AI: For Agentforce in particular, “explainability” is a big topic. Being able to point to how the data was aggregated, where it was sourced, and what transformations were applied fosters confidence across the organization.
Realistically, you don’t need to adopt a heavyweight tool if that’s not your style—but you do need some method of capturing each data pipeline, identity resolution rule, or agent skill. It might be visual flow diagrams, metadata dictionaries, or living documentation inside a repository. The key is to make it accessible, accurate, and kept up to date.
Real-World Examples of Smart Data Strategy
Salesforce’s Own Customer Zero Case
Salesforce leads by example with “Agentforce for SDRs.” They began with simple AI skills to handle routine lead nurturing. However, the real story is how they spent considerable time refining the underlying data. By making sure accounts, contacts, and hierarchies were consistent and up to date, they set Agentforce up for success. From there, it became exponentially easier to:
- Reuse Existing Data: The same curated customer data powers both a website AI agent and an SDR agent reaching out via email.
- Enable Autonomous Responses: Basic product Q&A is handled by the agent, freeing reps to handle more complex conversations.
- Stay Compliant: If a customer wants to opt out of communications, Agentforce flags the record and updates the preference in real time—no extra work from the sales team required.
The Contact Value Score
Another illuminating example comes from a team that wanted a “Contact Value Score” to see how engaged individual customers are across various touchpoints—marketing campaigns, product usage, support cases, and training completions. By funneling these data streams into Data Cloud and applying a calculated insight, they achieved a holistic snapshot per contact. This single metric then enabled more precise targeting: if a contact had high activity levels, they’d receive one kind of email or sales follow-up; if they were under-engaged, they’d get a different nudge. The key was weaving data from four or five separate systems into a single source of truth before letting any AI or automation do its magic.
Final Thoughts: Data as Your North Star
Ultimately, implementing Data Cloud or Agentforce is akin to building a modern, dynamic house: if your foundation (data strategy) is fragile, no amount of shiny AI “fixtures” will keep it standing. By making thoughtful choices around architecture, cost, governance, and data quality, you lay the groundwork for not just one successful project, but a series of scalable, AI-driven initiatives.
Here’s the bottom line to keep in mind:
- Define your use cases so Data Cloud solves real problems rather than serving as a mere technology showcase.
- Plan meticulously—embrace the notion of “9 parts planning, 1 part building.”
- Model your usage costs carefully. The consumption-based billing is very different from the typical SaaS user license.
- Document thoroughly—architecture diagrams, data flow maps, and data dictionaries ensure each phase of your project is robust and future-proof.
With this approach, you’re not just implementing a fancy new data tool; you’re setting your organization on a path toward true data-driven transformation, one where AI and human teams collaborate seamlessly to delight your customers and grow your business.
Building Trust Starts With A Conversation.
Ready to Take the Next Step?
If you and your team are considering a Data Cloud project, have questions, or simply want to learn more about best practices, please reach out and connect with us today. We’d love to talk through your unique needs, share our insights, and help you launch a high-impact data strategy that sets the stage for successful Agentforce or AI-driven initiatives. Don’t hesitate to get in touch—we’d love to be in touch with you!